*ERRATUM: In Renauld2023, it is stated that this processed data F1 scores of up to 64%. However, unfortunately, the non-recovered bundles (here 2; CA and CP) were forgotten in this average, which now reach up to 58%. Correct scores are now shown on figure below.
We offer here examples of tractograms created on carefully preprocessed data, as discussed in Renauld2023. The preprocessing choices were made after the publication of the 2017 paper, with knowledge available from the analysis of the results of the initial submissions. For instance, this could be used as training set in a machine learning experiment, to avoid training on the ground truth data.
How to cite: Please visit the References page to cite us if you use this data.
Preprocessing steps
The general preprocessing steps were the default Tractoflow pipeline. See the description in the Tractoflow documentation. Data was run with an adapted script based on version 2.3.0. In summary:
- DWI: Second timepoint of the DWI was extracted, named rev_b0 and added to the input data to allow using the topup step. Preprocessing steps were: Brain extraction, DWI denoising, Eddy/Topup, N4, Cropping, Normalization, Resampling, Extraction of fODF shells, Computation of fODF metrics.
- T1: Denoising, N4, Resampling, Brain extraction, Croping, Registration on the DWI, Segmentation of tracking/seeding maps.
- Tractography: We used seeding from either the WM mask (npv 10) or the interface mask (npv 60), and tracking with both local tracking and PFT tracking algorithms.
- Post-processing: We filtered streamlines based on length (20-200mm) and on detection of loops using scilpy’s scripts.
Results
The processed data can be downloaded here.
We verified data quality by scoring the three tractograms with the ROI-based scoring system (Renauld2023), with previously unseen results.
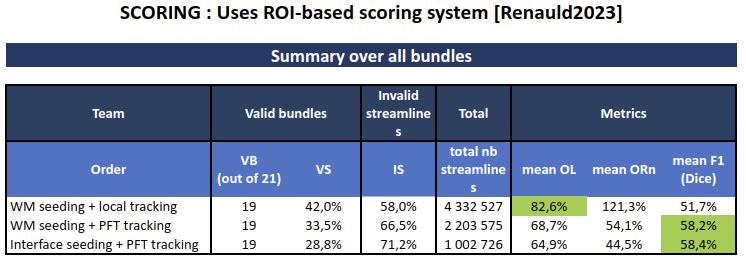